ECE Faculty Dario Pompili received a new supplement award from NSF
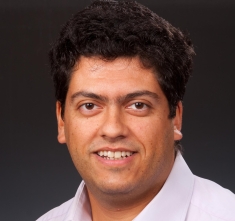
ECE Professor Dario Pompili received a $300K Supplement Award from the NSF as part of his on-going $500K Spectrum and Wireless Innovation enabled by Future Technologies (SWIFT) project titled "xl_NGRAN–Navigating Spectral Utilization, LTE/WiFi Coexistence, and Cost Tradeoffs in Next Gen Radio Access Networks through Cross-Layer Design".
This Supplement is awarded by the National Radio Dynamic Zones (NRDZ) program and will focus on NextG Spectrum Sharing for O-RAN-based Emergency Networking via Deep Multi-Agent Reinforcement Learning.
Overview
The Next radio Generation (NextG) of mobile networks, 6G and beyond, is expected to speed up the transition from monolithic and inflexible networks to agile and distributed networking elements that rely on “virtualization”, “softwarization”, openness, intelligent and yet fully interoperable Radio Access Network (RAN) components. Within the current virtual RAN (vRAN) and cloud RAN (C-RAN) concepts, spectrum sharing offers a natural solution to provide more efficient utilization of the spectrum by enabling different users or systems to share the same frequency bands dynamically. However, the traditional RAN framework involves large vendors creating proprietary hardware and software. As a result, in 2018, the O-RAN alliance was formed to realize the NextG cellular networks with flexible multi-vendor network infrastructure to the telecom operators. By deploying O-RAN, the network operators can significantly reduce the operational cost in a dense environment compared to vRAN and C-RAN. Due to the dynamic nature of spectrum policies and the unpredictability of unlicensed usage, spectrum sharing will require RANs to change their operational parameters intelligently and according to the current spectrum context. Although existing RANs do not allow for real-time reconfiguration, the fast-paced rise of the open RAN movement and of the O-RAN framework for NextG networks, where the hardware and software portions of the RAN are logically disaggregated, will allow seamless reconfiguration and optimization of the radio components.
Intellectual Merit
In light of the above, a Multi-Agent Reinforcement Learning (MARL)-based cross-layer communication framework, “RescueNet”, was developed by the PI for self-adaptation of nodes in emergency networks. The use of mission policies, which vary over time and space, enabled graceful degradation in the Quality of Service (QoS) of the incumbent networks (only when necessary) based on mission policy specifications. Specifically, RescueNet was designed to solve the spectrum-sharing problem in emergency networking; however, this framework has limitations. Firstly, it converges slowly and requires a large amount of data to train, indicating that the RL agents need to interact with the environment extensively, which is time and bandwidth consuming. Secondly, RescueNet adopts Q-learning as the basic policy. Nonetheless, Q-learning cannot handle continuous action spaces, which poses additional constraints to the applications that RescueNet can handle.
To deal with these limitations, as part of this Supplement Award, the PI will perform two research tasks. In Task 1, the PI will focus on adopting MARL for emergency networking in O-RAN architecture. Specifically, in Task 1.A, the PI proposes knowledge sharing among agents to aid RescueNet in terms of convergence rate. Experienced agents will transfer their knowledge to new agents so that the new agents can avoid starting from scratch. In Task 1.B, the PI proposes to combine RescueNet with O-RAN leveraging its Near-Real-Time (NRT) Radio Intelligent Controller (RIC), and proposes intelligent spectrum management unit and policy decision unit for NRT decision making. Then, in Task 2, the PI will propose deep Hierarchical Multi-Agent Actor-Critic (HMAAC) by adding a high-level policy for coordination to the Multi-Agent Actor Critic (MAAC) framework to tackle the convergence rate and action space problems. With the help of deep neural networks and hierarchical structures, coordination and knowledge sharing will become more efficient. In Task 2.A, the PI will propose decentralized HMAAC, where the high-level policy is assigned to multiple agents to avoid single-point-failure. Finally, in Task 2.B, the PI will propose to extend HMAAC to the multi-team scenario where multiple teams can cooperate together. In this setting, another hierarchy will be added to HMAAC to coordinate among teams; furthermore, a communication reward will be included to encourage the agents to maintain communication.
More info can be found here: https://www.nsf.gov/awardsearch/showAward?AWD_ID=2030101&HistoricalAwards=false
Congratulations to Dario!